from lec_utils import *
def show_grouping_animation():
src = "https://docs.google.com/presentation/d/1tBaFyHseIGsX5wmE3BdNLeVHnKksQtpzLhHge8Tzly0/embed?start=false&loop=false&delayms=60000&rm=minimal"
width = 960
height = 509
display(IFrame(src, width, height))
Announcements 📣¶
- Homework 2 is due on Thursday.
Post on Ed or
come to Office Hours for help! We're using a queue for office hours now – access it from practicaldsc.org/calendar.
study.practicaldsc.org contains our discussion worksheets (and solutions), which are made up of old exam problems. Use these problems to build your theoretical understanding of the material!
Homework 1 scores are available on Gradescope.
Agenda¶
- Recap: Querying.
- Adding and modifying columns.
pandas
andnumpy
.- Introduction to the
groupby
method. groupby
's inner workings.
Remember to follow along in lecture by accessing the "blank" lecture notebook in our public GitHub repository.
Question 🤔 (Answer at practicaldsc.org/q)
Remember that you can always ask questions anonymously at the link above!
Run the cell below to load in our dataset.
dogs = pd.read_csv('data/dogs43.csv').set_index('breed')
dogs.head()
kind | lifetime_cost | longevity | size | weight | height | |
---|---|---|---|---|---|---|
breed | ||||||
Brittany | sporting | 22589.0 | 12.92 | medium | 35.0 | 19.0 |
Cairn Terrier | terrier | 21992.0 | 13.84 | small | 14.0 | 10.0 |
English Cocker Spaniel | sporting | 18993.0 | 11.66 | medium | 30.0 | 16.0 |
Cocker Spaniel | sporting | 24330.0 | 12.50 | small | 25.0 | 14.5 |
Shetland Sheepdog | herding | 21006.0 | 12.53 | small | 22.0 | 14.5 |
Recap: Querying¶
- Querying is the act selecting rows in a DataFrame that satisfy certain condition(s).
We sometimes call this "filtering."
- Example: How many breeds live to be over 10 years old?
dogs[dogs['longevity'] > 10].shape[0]
32
- Example: Among all breeds with
'Retriever'
in the same, which is the second tallest?
Remember, we like to format our code this way when writing long, hard-to-read lines.
(
dogs.loc[dogs.index.str.contains('Retriever'), 'height']
.sort_values(ascending=False)
.iloc[1]
)
23.0
- What is the distribution of breeds
'size'
s among'sporting'
and'working'
breeds?
Remember, you need parentheses around each condition. Also, you must use the bitwise operators&
and|
instead of the standardand
andor
keywords, as we saw in Lecture 3.
(
dogs.loc[(dogs['kind'] == 'sporting') | (dogs['kind'] == 'working'), 'size']
.value_counts()
)
size large 11 medium 7 small 1 Name: count, dtype: int64
# Equivalent to the above!
(
dogs.loc[dogs['kind'].isin(['sporting', 'working']), 'size']
.value_counts()
)
size large 11 medium 7 small 1 Name: count, dtype: int64
- Show me all rows for
'medium'
-sized dogs.
dogs[dogs['size'] == 'medium']
kind | lifetime_cost | longevity | size | weight | height | |
---|---|---|---|---|---|---|
breed | ||||||
Brittany | sporting | 22589.0 | 12.92 | medium | 35.0 | 19.00 |
English Cocker Spaniel | sporting | 18993.0 | 11.66 | medium | 30.0 | 16.00 |
Siberian Husky | working | 22049.0 | 12.58 | medium | 47.5 | 21.75 |
... | ... | ... | ... | ... | ... | ... |
Clumber Spaniel | sporting | 18084.0 | 10.00 | medium | 70.0 | 18.50 |
Kerry Blue Terrier | terrier | 17240.0 | 9.40 | medium | 36.5 | 18.50 |
Bull Terrier | terrier | 18490.0 | 10.21 | medium | 60.0 | 21.50 |
12 rows × 6 columns
- Show me all rows for
'Golden Retriever'
s.
Note that because we set the index to'breed'
earlier, we can select rows based on dog breeds without having to query. If'breed'
was instead a column, then we'd need to query to access information about a particular breed.
dogs.loc['Golden Retriever']
kind sporting lifetime_cost 21447.0 longevity 12.04 size medium weight 60.0 height 22.75 Name: Golden Retriever, dtype: object
Aside: Reference Slides
- Moving forward, I'm going to try and spend a bit less time on syntax and a bit more time on conceptual problem-solving.
- So, in each lecture, some slides will be called "Reference Slides".
- We will skip these slides during live lecture, but they'll be present in the posted lecture notebooks, so that you can look at them when working on activities in class and on discussion and homework problems.
The material in them is in-scope.
- Reference slides will appear with red headers, like this one.
There's a Reference Slide right after this slide, about the DataFramequery
method!
The DataFrame query
method is a convenient way to query, since you don't need parentheses and you can use the and
and or
keywords.
dogs
kind | lifetime_cost | longevity | size | weight | height | |
---|---|---|---|---|---|---|
breed | ||||||
Brittany | sporting | 22589.0 | 12.92 | medium | 35.0 | 19.0 |
Cairn Terrier | terrier | 21992.0 | 13.84 | small | 14.0 | 10.0 |
English Cocker Spaniel | sporting | 18993.0 | 11.66 | medium | 30.0 | 16.0 |
... | ... | ... | ... | ... | ... | ... |
Bullmastiff | working | 13936.0 | 7.57 | large | 115.0 | 25.5 |
Mastiff | working | 13581.0 | 6.50 | large | 175.0 | 30.0 |
Saint Bernard | working | 20022.0 | 7.78 | large | 155.0 | 26.5 |
43 rows × 6 columns
dogs.query('weight < 20 and kind == "terrier"')
kind | lifetime_cost | longevity | size | weight | height | |
---|---|---|---|---|---|---|
breed | ||||||
Cairn Terrier | terrier | 21992.0 | 13.84 | small | 14.0 | 10.0 |
Miniature Schnauzer | terrier | 20087.0 | 11.81 | small | 15.5 | 13.0 |
Norfolk Terrier | terrier | 24308.0 | 13.07 | small | 12.0 | 9.5 |
dogs.query('kind in ["sporting", "terrier"] and lifetime_cost < 20000')
kind | lifetime_cost | longevity | size | weight | height | |
---|---|---|---|---|---|---|
breed | ||||||
English Cocker Spaniel | sporting | 18993.0 | 11.66 | medium | 30.0 | 16.0 |
Chesapeake Bay Retriever | sporting | 16697.0 | 9.48 | large | 67.5 | 23.5 |
Gordon Setter | sporting | 19605.0 | 11.10 | large | 62.5 | 25.0 |
Clumber Spaniel | sporting | 18084.0 | 10.00 | medium | 70.0 | 18.5 |
Scottish Terrier | terrier | 17525.0 | 10.69 | small | 20.0 | 10.0 |
Kerry Blue Terrier | terrier | 17240.0 | 9.40 | medium | 36.5 | 18.5 |
Bull Terrier | terrier | 18490.0 | 10.21 | medium | 60.0 | 21.5 |
jack = pd.DataFrame({1: ['fee', 'fi'],
'1': ['fo', 'fum']})
jack
1 | 1 | |
---|---|---|
0 | fee | fo |
1 | fi | fum |
For each of the following pieces of code, predict what the output will be. Then, uncomment the line of code and see for yourself. We won't cover these in class, but you should try them out yourself. Here's a Pandas Tutor link to visualize these!
# jack[1]
# jack[[1]]
# jack['1']
# jack[[1, 1]]
# jack.loc[1]
# jack.loc[jack[1] == 'fo']
# jack[1, ['1', 1]]
# jack.loc[1,1]
Adding and modifying columns¶
Adding and modifying columns, using a copy¶
- To add a new column to a DataFrame, use the
assign
method.
To change the values in a column, add a new column with the same name as the existing column.
- Like most
pandas
methods,assign
returns a new DataFrame.- Pro ✅: This doesn't inadvertently change any existing variables.
- Con ❌: It is not very space efficient, as it creates a new copy each time it is called.
dogs.assign(cost_per_year=dogs['lifetime_cost'] / dogs['longevity'])
kind | lifetime_cost | longevity | size | weight | height | cost_per_year | |
---|---|---|---|---|---|---|---|
breed | |||||||
Brittany | sporting | 22589.0 | 12.92 | medium | 35.0 | 19.0 | 1748.37 |
Cairn Terrier | terrier | 21992.0 | 13.84 | small | 14.0 | 10.0 | 1589.02 |
English Cocker Spaniel | sporting | 18993.0 | 11.66 | medium | 30.0 | 16.0 | 1628.90 |
... | ... | ... | ... | ... | ... | ... | ... |
Bullmastiff | working | 13936.0 | 7.57 | large | 115.0 | 25.5 | 1840.95 |
Mastiff | working | 13581.0 | 6.50 | large | 175.0 | 30.0 | 2089.38 |
Saint Bernard | working | 20022.0 | 7.78 | large | 155.0 | 26.5 | 2573.52 |
43 rows × 7 columns
dogs
kind | lifetime_cost | longevity | size | weight | height | |
---|---|---|---|---|---|---|
breed | ||||||
Brittany | sporting | 22589.0 | 12.92 | medium | 35.0 | 19.0 |
Cairn Terrier | terrier | 21992.0 | 13.84 | small | 14.0 | 10.0 |
English Cocker Spaniel | sporting | 18993.0 | 11.66 | medium | 30.0 | 16.0 |
... | ... | ... | ... | ... | ... | ... |
Bullmastiff | working | 13936.0 | 7.57 | large | 115.0 | 25.5 |
Mastiff | working | 13581.0 | 6.50 | large | 175.0 | 30.0 |
Saint Bernard | working | 20022.0 | 7.78 | large | 155.0 | 26.5 |
43 rows × 6 columns
You can also use assign
when the desired column name has spaces (and other special characters) by unpacking a dictionary:
dogs.assign(**{'cost per year 💵': dogs['lifetime_cost'] / dogs['longevity']})
kind | lifetime_cost | longevity | size | weight | height | cost per year 💵 | |
---|---|---|---|---|---|---|---|
breed | |||||||
Brittany | sporting | 22589.0 | 12.92 | medium | 35.0 | 19.0 | 1748.37 |
Cairn Terrier | terrier | 21992.0 | 13.84 | small | 14.0 | 10.0 | 1589.02 |
English Cocker Spaniel | sporting | 18993.0 | 11.66 | medium | 30.0 | 16.0 | 1628.90 |
... | ... | ... | ... | ... | ... | ... | ... |
Bullmastiff | working | 13936.0 | 7.57 | large | 115.0 | 25.5 | 1840.95 |
Mastiff | working | 13581.0 | 6.50 | large | 175.0 | 30.0 | 2089.38 |
Saint Bernard | working | 20022.0 | 7.78 | large | 155.0 | 26.5 | 2573.52 |
43 rows × 7 columns
Adding and modifying columns, in-place¶
- You can assign a new column to a DataFrame in-place using
[]
.
This works like dictionary assignment. Using[]
modifies the underlying DataFrame, unlikeassign
, which returns a new DataFrame.
- This is the more "common" way of adding/modifying columns.
Exercise caution when using this approach, since it is destructive – it changes the values of existing variables.
# By default, .copy() returns a deep copy of the object it is called on,
# meaning that if you change the copy, the original remains unmodified.
dogs_copy = dogs.copy()
dogs_copy.head(2)
kind | lifetime_cost | longevity | size | weight | height | |
---|---|---|---|---|---|---|
breed | ||||||
Brittany | sporting | 22589.0 | 12.92 | medium | 35.0 | 19.0 |
Cairn Terrier | terrier | 21992.0 | 13.84 | small | 14.0 | 10.0 |
dogs_copy['cost_per_year'] = dogs_copy['lifetime_cost'] / dogs_copy['longevity']
dogs_copy
kind | lifetime_cost | longevity | size | weight | height | cost_per_year | |
---|---|---|---|---|---|---|---|
breed | |||||||
Brittany | sporting | 22589.0 | 12.92 | medium | 35.0 | 19.0 | 1748.37 |
Cairn Terrier | terrier | 21992.0 | 13.84 | small | 14.0 | 10.0 | 1589.02 |
English Cocker Spaniel | sporting | 18993.0 | 11.66 | medium | 30.0 | 16.0 | 1628.90 |
... | ... | ... | ... | ... | ... | ... | ... |
Bullmastiff | working | 13936.0 | 7.57 | large | 115.0 | 25.5 | 1840.95 |
Mastiff | working | 13581.0 | 6.50 | large | 175.0 | 30.0 | 2089.38 |
Saint Bernard | working | 20022.0 | 7.78 | large | 155.0 | 26.5 | 2573.52 |
43 rows × 7 columns
- Note that we never reassigned
dogs_copy
in the cell above – that is, we never wrotedogs_copy = ...
– though it was still modified.
Mutability¶
- DataFrames, like lists, arrays, and dictionaries, are mutable. As we saw in Lecture 2, this means they can be modified in-place after creation.
- Not only does this explain the behavior on the previous slide, but it also explains the following:
dogs_copy
kind | lifetime_cost | longevity | size | weight | height | cost_per_year | |
---|---|---|---|---|---|---|---|
breed | |||||||
Brittany | sporting | 22589.0 | 12.92 | medium | 35.0 | 19.0 | 1748.37 |
Cairn Terrier | terrier | 21992.0 | 13.84 | small | 14.0 | 10.0 | 1589.02 |
English Cocker Spaniel | sporting | 18993.0 | 11.66 | medium | 30.0 | 16.0 | 1628.90 |
... | ... | ... | ... | ... | ... | ... | ... |
Bullmastiff | working | 13936.0 | 7.57 | large | 115.0 | 25.5 | 1840.95 |
Mastiff | working | 13581.0 | 6.50 | large | 175.0 | 30.0 | 2089.38 |
Saint Bernard | working | 20022.0 | 7.78 | large | 155.0 | 26.5 | 2573.52 |
43 rows × 7 columns
def cost_in_thousands():
dogs_copy['lifetime_cost'] = dogs_copy['lifetime_cost'] / 1000
# What happens when we run this twice? Three times?
cost_in_thousands()
dogs_copy
kind | lifetime_cost | longevity | size | weight | height | cost_per_year | |
---|---|---|---|---|---|---|---|
breed | |||||||
Brittany | sporting | 22.59 | 12.92 | medium | 35.0 | 19.0 | 1748.37 |
Cairn Terrier | terrier | 21.99 | 13.84 | small | 14.0 | 10.0 | 1589.02 |
English Cocker Spaniel | sporting | 18.99 | 11.66 | medium | 30.0 | 16.0 | 1628.90 |
... | ... | ... | ... | ... | ... | ... | ... |
Bullmastiff | working | 13.94 | 7.57 | large | 115.0 | 25.5 | 1840.95 |
Mastiff | working | 13.58 | 6.50 | large | 175.0 | 30.0 | 2089.38 |
Saint Bernard | working | 20.02 | 7.78 | large | 155.0 | 26.5 | 2573.52 |
43 rows × 7 columns
⚠️ Warning: Avoid mutation when possible!¶
- Note that
dogs_copy
was modified, even though we didn't reassign it! These unintended consequences can influence the behavior of test cases on homeworks, among other things!
- To avoid this, it's a good idea to avoid mutation when possible. If you must use mutation, include
df = df.copy()
as the first line in functions that take DataFrames as input.
Question 🤔 (Answer at practicaldsc.org/q)
Remember that you can always ask questions anonymously at the link above!
pandas
and numpy
¶
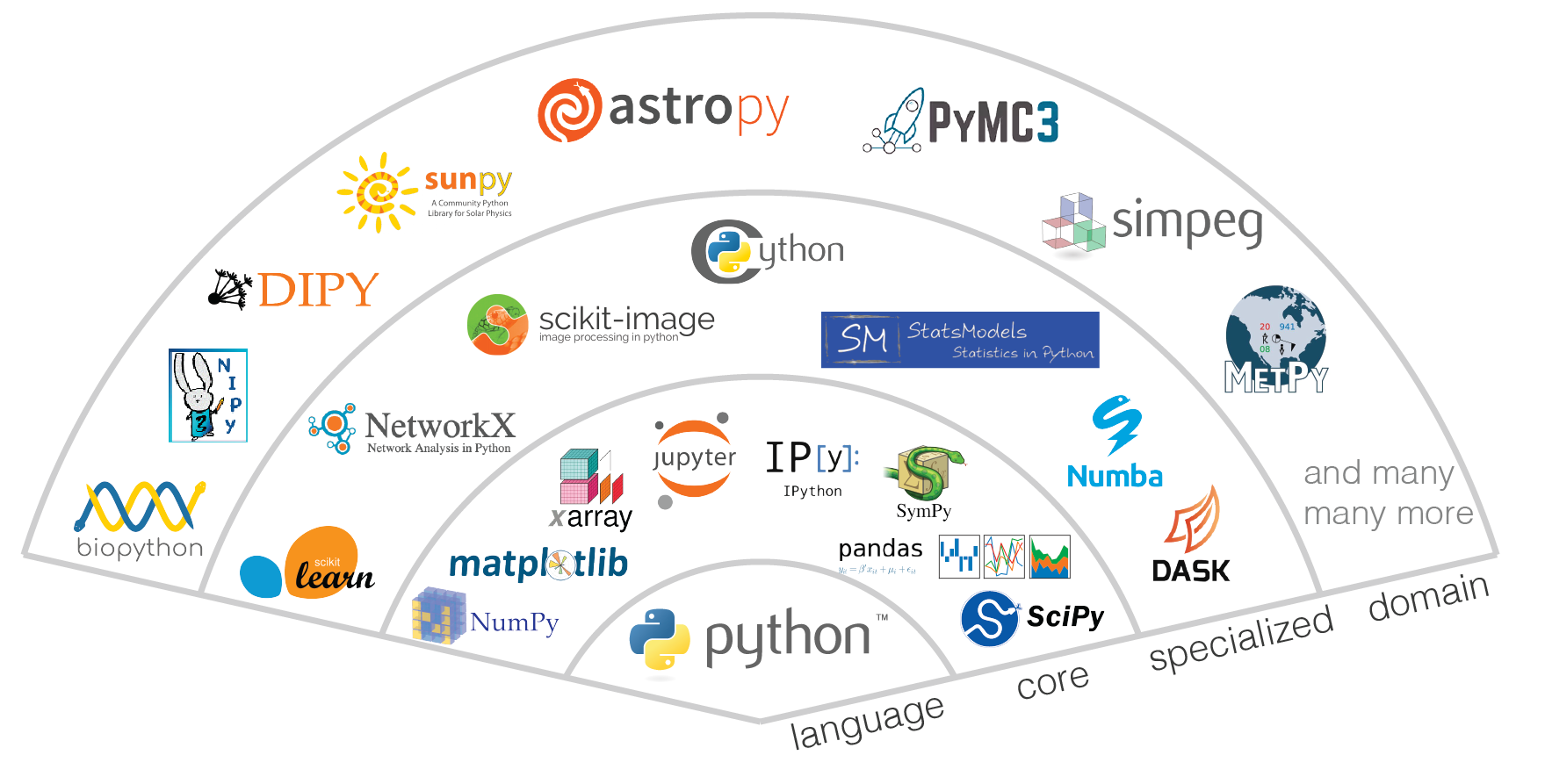
pandas
is built upon numpy
!¶
- A Series in
pandas
is anumpy
array with an index.
- A DataFrame is like a dictionary of columns, each of which is a
numpy
array.
- Many operations in
pandas
are fast because they usenumpy
's implementations, which are written in fast, compiled languages like C.
- If you need to access the array underlying a DataFrame or Series, use the
to_numpy
method.
dogs['lifetime_cost']
breed Brittany 22589.0 Cairn Terrier 21992.0 English Cocker Spaniel 18993.0 ... Bullmastiff 13936.0 Mastiff 13581.0 Saint Bernard 20022.0 Name: lifetime_cost, Length: 43, dtype: float64
dogs['lifetime_cost'].to_numpy()
array([22589., 21992., 18993., ..., 13936., 13581., 20022.])
- Each Series (column) has a
numpy
data type, which refers to the type of the values stored within. Access it using thedtypes
attribute.
- A column's data type determines which operations can be applied to it.
pandas
tries to guess the correct data types for a given DataFrame, and is often wrong.- This can lead to incorrect calculations and poor memory/time performance.
- As a result, you will often need to explicitly convert between data types.
dogs
kind | lifetime_cost | longevity | size | weight | height | |
---|---|---|---|---|---|---|
breed | ||||||
Brittany | sporting | 22589.0 | 12.92 | medium | 35.0 | 19.0 |
Cairn Terrier | terrier | 21992.0 | 13.84 | small | 14.0 | 10.0 |
English Cocker Spaniel | sporting | 18993.0 | 11.66 | medium | 30.0 | 16.0 |
... | ... | ... | ... | ... | ... | ... |
Bullmastiff | working | 13936.0 | 7.57 | large | 115.0 | 25.5 |
Mastiff | working | 13581.0 | 6.50 | large | 175.0 | 30.0 |
Saint Bernard | working | 20022.0 | 7.78 | large | 155.0 | 26.5 |
43 rows × 6 columns
dogs.dtypes
kind object lifetime_cost float64 longevity float64 size object weight float64 height float64 dtype: object
- Notice that Python
str
types areobject
types innumpy
andpandas
.
Pandas dtype | Python type | NumPy type | SQL type | Usage |
---|---|---|---|---|
int64 | int | int_, int8,...,int64, uint8,...,uint64 | INT, BIGINT | Integer numbers |
float64 | float | float_, float16, float32, float64 | FLOAT | Floating point numbers |
bool | bool | bool_ | BOOL | True/False values |
datetime64 or Timestamp | datetime.datetime | datetime64 | DATETIME | Date and time values |
timedelta64 or Timedelta | datetime.timedelta | timedelta64 | NA | Differences between two datetimes |
category | NA | NA | ENUM | Finite list of text values |
object | str | string, unicode | NA | Text |
object | NA | object | NA | Mixed types |
- This article details how
pandas
stores different data types under the hood.
- This article explains how
numpy
/pandas
int64
operations differ from vanillaint
operations.
- You can change the data type of a Series using the
.astype
Series method.
- For example, we can change the data type of the
'lifetime_cost'
column indogs
to beuint32
:
dogs
kind | lifetime_cost | longevity | size | weight | height | |
---|---|---|---|---|---|---|
breed | ||||||
Brittany | sporting | 22589.0 | 12.92 | medium | 35.0 | 19.0 |
Cairn Terrier | terrier | 21992.0 | 13.84 | small | 14.0 | 10.0 |
English Cocker Spaniel | sporting | 18993.0 | 11.66 | medium | 30.0 | 16.0 |
... | ... | ... | ... | ... | ... | ... |
Bullmastiff | working | 13936.0 | 7.57 | large | 115.0 | 25.5 |
Mastiff | working | 13581.0 | 6.50 | large | 175.0 | 30.0 |
Saint Bernard | working | 20022.0 | 7.78 | large | 155.0 | 26.5 |
43 rows × 6 columns
# Gives the types as well as the space taken up by the DataFrame.
dogs.info()
<class 'pandas.core.frame.DataFrame'> Index: 43 entries, Brittany to Saint Bernard Data columns (total 6 columns): # Column Non-Null Count Dtype --- ------ -------------- ----- 0 kind 43 non-null object 1 lifetime_cost 43 non-null float64 2 longevity 43 non-null float64 3 size 43 non-null object 4 weight 43 non-null float64 5 height 43 non-null float64 dtypes: float64(4), object(2) memory usage: 3.4+ KB
dogs['lifetime_cost'] = dogs['lifetime_cost'].astype('uint32')
- Now, the DataFrame takes up less space! This may be insignificant in our DataFrame, but makes a difference when working with larger datasets.
dogs.info()
<class 'pandas.core.frame.DataFrame'> Index: 43 entries, Brittany to Saint Bernard Data columns (total 6 columns): # Column Non-Null Count Dtype --- ------ -------------- ----- 0 kind 43 non-null object 1 lifetime_cost 43 non-null uint32 2 longevity 43 non-null float64 3 size 43 non-null object 4 weight 43 non-null float64 5 height 43 non-null float64 dtypes: float64(3), object(2), uint32(1) memory usage: 3.2+ KB
Usually, we prefer to set the correct dtypes in read_csv
, since it can help pandas
load in files more quickly:
dogs_new = pd.read_csv('data/dogs43.csv', dtype={'lifetime_cost': 'uint32'})
dogs_new
breed | kind | lifetime_cost | longevity | size | weight | height | |
---|---|---|---|---|---|---|---|
0 | Brittany | sporting | 22589 | 12.92 | medium | 35.0 | 19.0 |
1 | Cairn Terrier | terrier | 21992 | 13.84 | small | 14.0 | 10.0 |
2 | English Cocker Spaniel | sporting | 18993 | 11.66 | medium | 30.0 | 16.0 |
... | ... | ... | ... | ... | ... | ... | ... |
40 | Bullmastiff | working | 13936 | 7.57 | large | 115.0 | 25.5 |
41 | Mastiff | working | 13581 | 6.50 | large | 175.0 | 30.0 |
42 | Saint Bernard | working | 20022 | 7.78 | large | 155.0 | 26.5 |
43 rows × 7 columns
dogs_new.dtypes
breed object kind object lifetime_cost uint32 longevity float64 size object weight float64 height float64 dtype: object
Axes¶
- The rows and columns of a DataFrame are both stored as Series.
- The axis specifies the direction of a slice of a DataFrame.
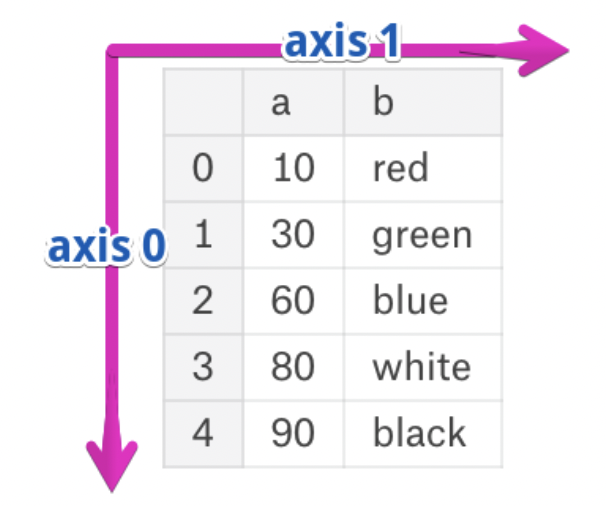
- Axis 0 refers to the rows and axis 1 refers to the columns.
These are the same axes definitions that 2Dnumpy
arrays have!
DataFrame methods with axis
¶
- Many Series methods work on DataFrames.
- In such cases, the DataFrame method usually applies the Series method to every row or column.
- Many of these methods accept an
axis
argument; the default is usuallyaxis=0
.
dogs
kind | lifetime_cost | longevity | size | weight | height | |
---|---|---|---|---|---|---|
breed | ||||||
Brittany | sporting | 22589 | 12.92 | medium | 35.0 | 19.0 |
Cairn Terrier | terrier | 21992 | 13.84 | small | 14.0 | 10.0 |
English Cocker Spaniel | sporting | 18993 | 11.66 | medium | 30.0 | 16.0 |
... | ... | ... | ... | ... | ... | ... |
Bullmastiff | working | 13936 | 7.57 | large | 115.0 | 25.5 |
Mastiff | working | 13581 | 6.50 | large | 175.0 | 30.0 |
Saint Bernard | working | 20022 | 7.78 | large | 155.0 | 26.5 |
43 rows × 6 columns
# Max element in each column.
dogs.max()
kind working lifetime_cost 26686 longevity 16.5 size small weight 175.0 height 30.0 dtype: object
# Max element in each row – a little nonsensical, since the values in each column are on different scales.
# Note that we had to select the numeric columns first.
dogs[['lifetime_cost', 'longevity', 'weight', 'height']].max(axis=1)
breed Brittany 22589.0 Cairn Terrier 21992.0 English Cocker Spaniel 18993.0 ... Bullmastiff 13936.0 Mastiff 13581.0 Saint Bernard 20022.0 Length: 43, dtype: float64
# The number of unique values in each column.
dogs.nunique()
kind 7 lifetime_cost 43 longevity 40 size 3 weight 37 height 30 dtype: int64
# describe doesn't accept an axis argument; it works on every numeric column in the DataFrame it is called on.
dogs.describe()
lifetime_cost | longevity | weight | height | |
---|---|---|---|---|
count | 43.00 | 43.00 | 43.00 | 43.00 |
mean | 20532.84 | 11.34 | 49.35 | 18.34 |
std | 3290.78 | 2.05 | 39.42 | 6.83 |
... | ... | ... | ... | ... |
50% | 21006.00 | 11.81 | 36.50 | 18.50 |
75% | 22072.50 | 12.52 | 67.50 | 25.00 |
max | 26686.00 | 16.50 | 175.00 | 30.00 |
8 rows × 4 columns
Activity
Pick a dog breed that you personally like or know the name of. Then:
- Try to find a few other dog breeds that are similar in weight to yours in
all_dogs
. - Which similar breeds have the lowest and highest
'lifetime_cost'
?'intelligence_rank'
? - Are there any similar breeds that you haven't heard of before?
For fun, look up these dog breeds on the AKC website to see what they look like!
all_dogs = pd.read_csv('data/all_dogs.csv')
all_dogs
breed | group | datadog | popularity_all | ... | megarank | size | weight | height | |
---|---|---|---|---|---|---|---|---|---|
0 | Border Collie | herding | 3.64 | 45 | ... | 29.0 | medium | NaN | 20.0 |
1 | Border Terrier | terrier | 3.61 | 80 | ... | 1.0 | small | 13.5 | NaN |
2 | Brittany | sporting | 3.54 | 30 | ... | 11.0 | medium | 35.0 | 19.0 |
... | ... | ... | ... | ... | ... | ... | ... | ... | ... |
169 | Wire Fox Terrier | terrier | NaN | 100 | ... | NaN | small | 17.5 | 15.0 |
170 | Wirehaired Pointing Griffon | sporting | NaN | 92 | ... | NaN | medium | NaN | 22.0 |
171 | Xoloitzcuintli | non-sporting | NaN | 155 | ... | NaN | medium | NaN | 16.5 |
172 rows × 18 columns
# There's no "right answer" here; you're supposed to explore!
fav_weight = all_dogs.loc[all_dogs['breed'] == 'English Cocker Spaniel', 'weight'].iloc[0]
similar_weight = all_dogs[(all_dogs['weight'] >= fav_weight - 5) & (all_dogs['weight'] <= fav_weight + 5)]
similar_weight
breed | group | datadog | popularity_all | ... | megarank | size | weight | height | |
---|---|---|---|---|---|---|---|---|---|
2 | Brittany | sporting | 3.54 | 30 | ... | 11.0 | medium | 35.0 | 19.00 |
5 | English Cocker Spaniel | sporting | 3.33 | 63 | ... | 5.0 | medium | 30.0 | 16.00 |
6 | Cocker Spaniel | sporting | 3.30 | 27 | ... | 6.0 | small | 25.0 | 14.50 |
... | ... | ... | ... | ... | ... | ... | ... | ... | ... |
125 | Irish Terrier | terrier | NaN | 132 | ... | NaN | medium | 26.0 | 18.00 |
138 | Norwegian Buhund | herding | NaN | 165 | ... | NaN | medium | 33.0 | 17.25 |
159 | Soft-Coated Wheaten Terrier | terrier | NaN | 52 | ... | NaN | medium | 35.0 | 18.00 |
12 rows × 18 columns
similar_weight.sort_values('intelligence_rank')[['breed', 'lifetime_cost', 'intelligence_rank']]
breed | lifetime_cost | intelligence_rank | |
---|---|---|---|
57 | Pembroke Welsh Corgi | 23978.0 | 11.0 |
5 | English Cocker Spaniel | 18993.0 | 18.0 |
2 | Brittany | 22589.0 | 19.0 |
... | ... | ... | ... |
73 | French Bulldog | 17266.0 | 58.0 |
117 | Glen of Imaal Terrier | NaN | NaN |
138 | Norwegian Buhund | NaN | NaN |
12 rows × 3 columns
Introduction to the groupby
method¶
Example: Palmer Penguins¶
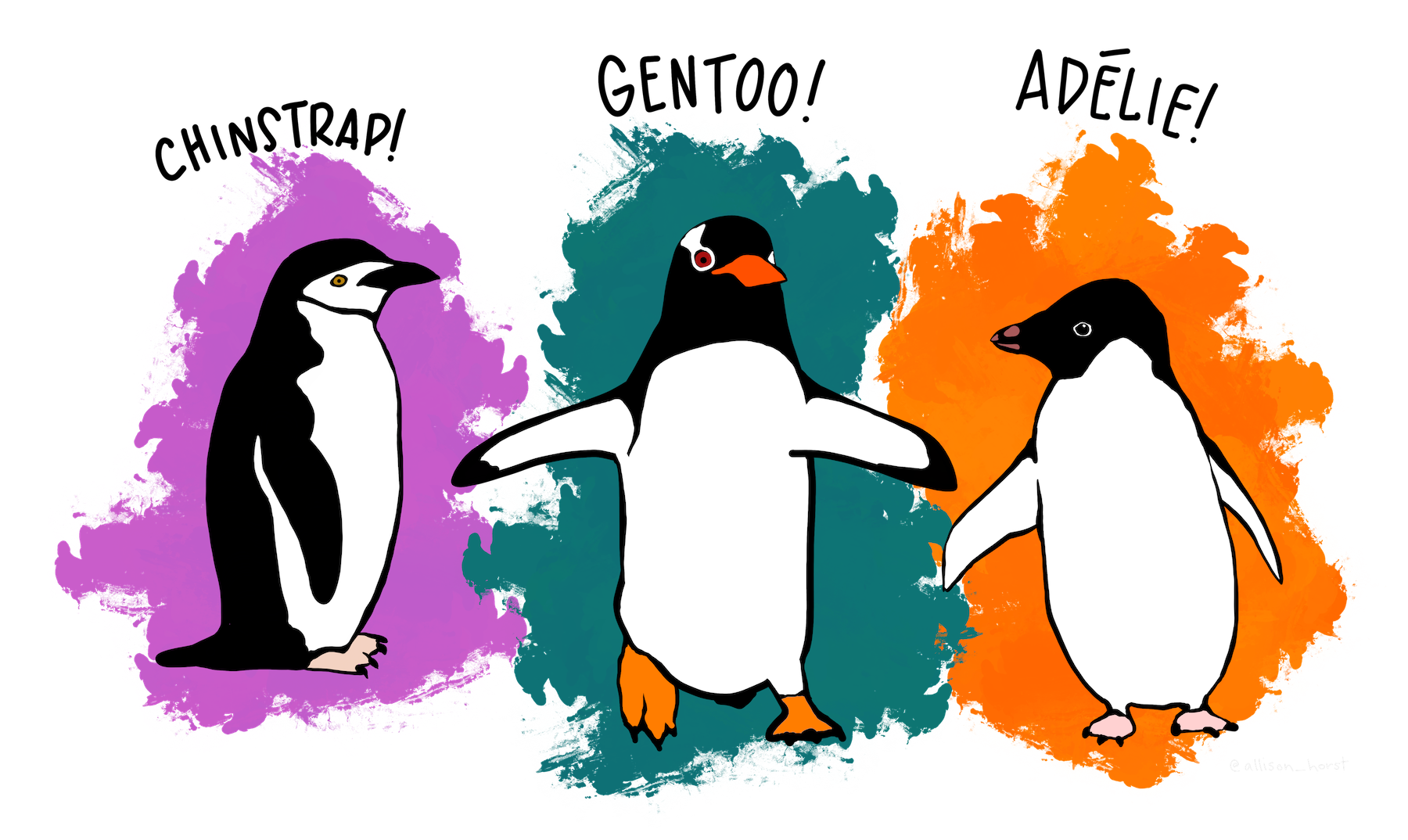
The dataset we'll work with for the rest of the lecture involves various measurements taken of three species of penguins in Antarctica.
IFrame('https://www.youtube-nocookie.com/embed/CCrNAHXUstU?si=-DntSyUNp5Kwitjm&start=11',
width=560, height=315)
Loading the data¶
penguins = sns.load_dataset('penguins').dropna().reset_index(drop=True)
penguins
species | island | bill_length_mm | bill_depth_mm | flipper_length_mm | body_mass_g | sex | |
---|---|---|---|---|---|---|---|
0 | Adelie | Torgersen | 39.1 | 18.7 | 181.0 | 3750.0 | Male |
1 | Adelie | Torgersen | 39.5 | 17.4 | 186.0 | 3800.0 | Female |
2 | Adelie | Torgersen | 40.3 | 18.0 | 195.0 | 3250.0 | Female |
... | ... | ... | ... | ... | ... | ... | ... |
330 | Gentoo | Biscoe | 50.4 | 15.7 | 222.0 | 5750.0 | Male |
331 | Gentoo | Biscoe | 45.2 | 14.8 | 212.0 | 5200.0 | Female |
332 | Gentoo | Biscoe | 49.9 | 16.1 | 213.0 | 5400.0 | Male |
333 rows × 7 columns
- Here, each row corresponds to a single penguin, and each column corresponds to a different attribute (or feature) we have for each penguin.
- Data formatted in this way is sometimes called tidy data.
Visualizing the data¶
penguins.plot(kind='scatter',
x='bill_length_mm',
y='body_mass_g',
color='species',
title='Body Mass vs. Bill Length')
Granularity¶
- Granularity refers to what each observation in a dataset represents.
- Fine: small details.
- Coarse: bigger picture.
- If you can control how your dataset is created, you should opt for finer granularity, i.e. for more detail.
- You can always remove details, but it's difficult to add detail that isn't already there.
- But obtaining fine-grained data can take more time/money.
- Today, we'll focus on how to remove details from fine-grained data, in order to help us understand bigger-picture trends in our data.
Aggregating¶
- Aggregating is the act of combining many values into a single value.
- What is the mean
'body_mass_g'
for all penguins?
penguins['body_mass_g'].mean()
4207.057057057057
- What is the mean
'body_mass_g'
for each'species'
?
A naïve approach to finding the mean 'body_mass_g'
per 'species'
¶
- First, we could identify all unique values in the
'species'
column.
penguins['species'].unique()
array(['Adelie', 'Chinstrap', 'Gentoo'], dtype=object)
- Then, for each
'species'
, we could:- Query for just that
'species'
. - Extract the
'body_mass_g'
column and use themean
method on it.
- Query for just that
penguins.loc[penguins['species'] == 'Adelie', 'body_mass_g'].mean()
3706.1643835616437
penguins.loc[penguins['species'] == 'Chinstrap', 'body_mass_g'].mean()
3733.0882352941176
penguins.loc[penguins['species'] == 'Gentoo', 'body_mass_g'].mean()
5092.436974789916
- We could use a
for
-loop, but remember, we want to avoid Pythonfor
-loops.
Grouping¶
- A better solution is to use the
groupby
method.
# To find the overall mean 'body_mass_g':
penguins['body_mass_g'].mean()
4207.057057057057
# To find the mean 'body_mass_g' for each 'species':
penguins.groupby('species')['body_mass_g'].mean()
species Adelie 3706.16 Chinstrap 3733.09 Gentoo 5092.44 Name: body_mass_g, dtype: float64
- Somehow, the
groupby
method computes what we're looking for in just one line. How?
- We'll work through the internals, but remember this: if you need to calculate something for each group, use
groupby
!
An illustrative example: Pets 🐱 🐶🐹¶
- Consider the DataFrame
pets
, shown below.
Species | Color | Weight | Age | |
---|---|---|---|---|
0 | dog | black | 40 | 5.0 |
1 | cat | golden | 15 | 8.0 |
2 | cat | black | 20 | 9.0 |
3 | dog | white | 80 | 2.0 |
4 | dog | golden | 25 | 0.5 |
5 | hamster | golden | 1 | 3.0 |
- Let's see what happens under the hood when we use the
groupby
method onpets
.
show_grouping_animation()
Let's try it out!¶
pets = pd.DataFrame().assign(
Species=['dog', 'cat', 'cat', 'dog', 'dog', 'hamster'],
Color=['black', 'golden', 'black', 'white', 'golden', 'golden'],
Weight=[40, 15, 20, 80, 25, 1],
Age=[5, 8, 9, 2, 0.5, 3]
)
pets
Species | Color | Weight | Age | |
---|---|---|---|---|
0 | dog | black | 40 | 5.0 |
1 | cat | golden | 15 | 8.0 |
2 | cat | black | 20 | 9.0 |
3 | dog | white | 80 | 2.0 |
4 | dog | golden | 25 | 0.5 |
5 | hamster | golden | 1 | 3.0 |
# Why does this error?
pets.groupby('Species').mean()
--------------------------------------------------------------------------- TypeError Traceback (most recent call last) File ~/miniforge3/envs/pds/lib/python3.10/site-packages/pandas/core/groupby/groupby.py:1870, in GroupBy._agg_py_fallback(self, how, values, ndim, alt) 1869 try: -> 1870 res_values = self.grouper.agg_series(ser, alt, preserve_dtype=True) 1871 except Exception as err: File ~/miniforge3/envs/pds/lib/python3.10/site-packages/pandas/core/groupby/ops.py:850, in BaseGrouper.agg_series(self, obj, func, preserve_dtype) 848 preserve_dtype = True --> 850 result = self._aggregate_series_pure_python(obj, func) 852 npvalues = lib.maybe_convert_objects(result, try_float=False) File ~/miniforge3/envs/pds/lib/python3.10/site-packages/pandas/core/groupby/ops.py:871, in BaseGrouper._aggregate_series_pure_python(self, obj, func) 870 for i, group in enumerate(splitter): --> 871 res = func(group) 872 res = extract_result(res) File ~/miniforge3/envs/pds/lib/python3.10/site-packages/pandas/core/groupby/groupby.py:2376, in GroupBy.mean.<locals>.<lambda>(x) 2373 else: 2374 result = self._cython_agg_general( 2375 "mean", -> 2376 alt=lambda x: Series(x).mean(numeric_only=numeric_only), 2377 numeric_only=numeric_only, 2378 ) 2379 return result.__finalize__(self.obj, method="groupby") File ~/miniforge3/envs/pds/lib/python3.10/site-packages/pandas/core/series.py:6226, in Series.mean(self, axis, skipna, numeric_only, **kwargs) 6218 @doc(make_doc("mean", ndim=1)) 6219 def mean( 6220 self, (...) 6224 **kwargs, 6225 ): -> 6226 return NDFrame.mean(self, axis, skipna, numeric_only, **kwargs) File ~/miniforge3/envs/pds/lib/python3.10/site-packages/pandas/core/generic.py:11969, in NDFrame.mean(self, axis, skipna, numeric_only, **kwargs) 11962 def mean( 11963 self, 11964 axis: Axis | None = 0, (...) 11967 **kwargs, 11968 ) -> Series | float: > 11969 return self._stat_function( 11970 "mean", nanops.nanmean, axis, skipna, numeric_only, **kwargs 11971 ) File ~/miniforge3/envs/pds/lib/python3.10/site-packages/pandas/core/generic.py:11926, in NDFrame._stat_function(self, name, func, axis, skipna, numeric_only, **kwargs) 11924 validate_bool_kwarg(skipna, "skipna", none_allowed=False) > 11926 return self._reduce( 11927 func, name=name, axis=axis, skipna=skipna, numeric_only=numeric_only 11928 ) File ~/miniforge3/envs/pds/lib/python3.10/site-packages/pandas/core/series.py:6134, in Series._reduce(self, op, name, axis, skipna, numeric_only, filter_type, **kwds) 6130 raise TypeError( 6131 f"Series.{name} does not allow {kwd_name}={numeric_only} " 6132 "with non-numeric dtypes." 6133 ) -> 6134 return op(delegate, skipna=skipna, **kwds) File ~/miniforge3/envs/pds/lib/python3.10/site-packages/pandas/core/nanops.py:147, in bottleneck_switch.__call__.<locals>.f(values, axis, skipna, **kwds) 146 else: --> 147 result = alt(values, axis=axis, skipna=skipna, **kwds) 149 return result File ~/miniforge3/envs/pds/lib/python3.10/site-packages/pandas/core/nanops.py:404, in _datetimelike_compat.<locals>.new_func(values, axis, skipna, mask, **kwargs) 402 mask = isna(values) --> 404 result = func(values, axis=axis, skipna=skipna, mask=mask, **kwargs) 406 if datetimelike: File ~/miniforge3/envs/pds/lib/python3.10/site-packages/pandas/core/nanops.py:720, in nanmean(values, axis, skipna, mask) 719 the_sum = values.sum(axis, dtype=dtype_sum) --> 720 the_sum = _ensure_numeric(the_sum) 722 if axis is not None and getattr(the_sum, "ndim", False): File ~/miniforge3/envs/pds/lib/python3.10/site-packages/pandas/core/nanops.py:1693, in _ensure_numeric(x) 1691 if isinstance(x, str): 1692 # GH#44008, GH#36703 avoid casting e.g. strings to numeric -> 1693 raise TypeError(f"Could not convert string '{x}' to numeric") 1694 try: TypeError: Could not convert string 'goldenblack' to numeric The above exception was the direct cause of the following exception: TypeError Traceback (most recent call last) Cell In[60], line 2 1 # Why does this error? ----> 2 pets.groupby('Species').mean() File ~/miniforge3/envs/pds/lib/python3.10/site-packages/pandas/core/groupby/groupby.py:2374, in GroupBy.mean(self, numeric_only, engine, engine_kwargs) 2367 return self._numba_agg_general( 2368 grouped_mean, 2369 executor.float_dtype_mapping, 2370 engine_kwargs, 2371 min_periods=0, 2372 ) 2373 else: -> 2374 result = self._cython_agg_general( 2375 "mean", 2376 alt=lambda x: Series(x).mean(numeric_only=numeric_only), 2377 numeric_only=numeric_only, 2378 ) 2379 return result.__finalize__(self.obj, method="groupby") File ~/miniforge3/envs/pds/lib/python3.10/site-packages/pandas/core/groupby/groupby.py:1925, in GroupBy._cython_agg_general(self, how, alt, numeric_only, min_count, **kwargs) 1922 result = self._agg_py_fallback(how, values, ndim=data.ndim, alt=alt) 1923 return result -> 1925 new_mgr = data.grouped_reduce(array_func) 1926 res = self._wrap_agged_manager(new_mgr) 1927 out = self._wrap_aggregated_output(res) File ~/miniforge3/envs/pds/lib/python3.10/site-packages/pandas/core/internals/managers.py:1428, in BlockManager.grouped_reduce(self, func) 1424 if blk.is_object: 1425 # split on object-dtype blocks bc some columns may raise 1426 # while others do not. 1427 for sb in blk._split(): -> 1428 applied = sb.apply(func) 1429 result_blocks = extend_blocks(applied, result_blocks) 1430 else: File ~/miniforge3/envs/pds/lib/python3.10/site-packages/pandas/core/internals/blocks.py:366, in Block.apply(self, func, **kwargs) 360 @final 361 def apply(self, func, **kwargs) -> list[Block]: 362 """ 363 apply the function to my values; return a block if we are not 364 one 365 """ --> 366 result = func(self.values, **kwargs) 368 result = maybe_coerce_values(result) 369 return self._split_op_result(result) File ~/miniforge3/envs/pds/lib/python3.10/site-packages/pandas/core/groupby/groupby.py:1922, in GroupBy._cython_agg_general.<locals>.array_func(values) 1919 else: 1920 return result -> 1922 result = self._agg_py_fallback(how, values, ndim=data.ndim, alt=alt) 1923 return result File ~/miniforge3/envs/pds/lib/python3.10/site-packages/pandas/core/groupby/groupby.py:1874, in GroupBy._agg_py_fallback(self, how, values, ndim, alt) 1872 msg = f"agg function failed [how->{how},dtype->{ser.dtype}]" 1873 # preserve the kind of exception that raised -> 1874 raise type(err)(msg) from err 1876 if ser.dtype == object: 1877 res_values = res_values.astype(object, copy=False) TypeError: agg function failed [how->mean,dtype->object]
pets.groupby('Species')[['Weight', 'Age']].mean()
Weight | Age | |
---|---|---|
Species | ||
cat | 17.50 | 8.5 |
dog | 48.33 | 2.5 |
hamster | 1.00 | 3.0 |
pets.groupby('Species').max()
Color | Weight | Age | |
---|---|---|---|
Species | |||
cat | golden | 20 | 9.0 |
dog | white | 80 | 5.0 |
hamster | golden | 1 | 3.0 |
It takes several steps to go from the original pets
DataFrame to this grouped DataFrame, but we don't get to see any of Python's inner workings, just the final output.
"Split-apply-combine" paradigm¶
- The
groupby
method involves three steps: split, apply, and combine.
This is the same terminology that thepandas
documentation uses.
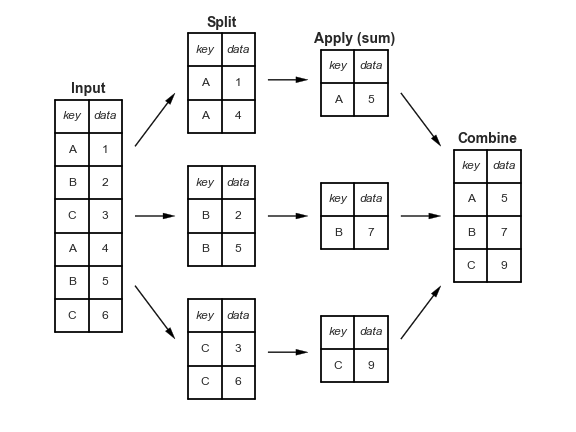
- Split breaks up and "groups" the rows of a DataFrame according to the specified key.
There is one "group" for every unique value of the key.
- Apply uses a function (e.g. aggregation, transformation, filtration) within the individual groups.
- Combine stitches the results of these operations into an output DataFrame.
- The split-apply-combine pattern can be parallelized to work on multiple computers or threads, by sending computations for each group to different processors.
More examples¶
Before we dive into the internals, let's look at a few more examples.
Activity
Which 'species'
has the highest median 'bill_length_mm'
?
(
penguins
.groupby('species')
['bill_length_mm']
.median()
.idxmax()
)
'Chinstrap'
(
penguins
.groupby('species')
['bill_length_mm']
.median()
.plot(kind='barh', title='Median Bill Length of Each Species')
)
Activity
What proportion of penguins of each 'species'
live on 'Dream'
island?
(
penguins
.assign(is_Dream=penguins['island'] == 'Dream')
.groupby('species')
['is_Dream']
.mean()
)
species Adelie 0.38 Chinstrap 1.00 Gentoo 0.00 Name: is_Dream, dtype: float64
groupby
's inner workings¶
How does groupby
actually work?¶
- We've just evaluated a few expressions of the following form.
penguins.groupby('species')['bill_length_mm'].mean()
species Adelie 38.82 Chinstrap 48.83 Gentoo 47.57 Name: bill_length_mm, dtype: float64
- There are three "building blocks"
in the above expression:
1. penguins.groupby('species')
.
First, we specify which column we want to group on.
1. ['bill_length_mm']
.
Then, we select the other relevant columns for our calculations.
1. .mean()
.
Finally, we use an aggregation method.
- Let's see what each block contributes to the output.
DataFrameGroupBy
objects¶
penguins.groupby('species')['bill_length_mm'].mean()
- If
df
is a DataFrame, thendf.groupby(key)
returns aDataFrameGroupBy
object.
This object represents the "split" in "split-apply-combine".
# Simplified DataFrame for demonstration:
penguins_small = penguins.iloc[[0, 150, 300, 1, 251, 151, 301], [0, 5, 6]]
penguins_small
species | body_mass_g | sex | |
---|---|---|---|
0 | Adelie | 3750.0 | Male |
150 | Chinstrap | 3725.0 | Male |
300 | Gentoo | 4875.0 | Female |
1 | Adelie | 3800.0 | Female |
251 | Gentoo | 4350.0 | Female |
151 | Chinstrap | 3950.0 | Female |
301 | Gentoo | 5550.0 | Male |
# Creates one group for each unique value in the species column.
penguins_small.groupby('species')
<pandas.core.groupby.generic.DataFrameGroupBy object at 0x309c06740>
DataFrameGroupBy
objects have agroups
attribute, which is a dictionary in which the keys are group names and the values are lists of row labels.
We won't actually use this, but it's helpful in understanding howgroupby
works under-the-hood.
penguins_small.groupby('species').groups
{'Adelie': [0, 1], 'Chinstrap': [150, 151], 'Gentoo': [300, 251, 301]}
Column extraction¶
penguins.groupby('species')['bill_length_mm'].mean()
- After creating a
DataFrameGroupBy
object, we typically select the relevant column(s) that we want to aggregate.
- The result is either a
SeriesGroupBy
orDataFrameGroupBy
object, depending on what's passed in.
penguins.groupby('species')['bill_length_mm']
<pandas.core.groupby.generic.SeriesGroupBy object at 0x168927400>
penguins.groupby('species')[['bill_length_mm', 'bill_depth_mm']]
<pandas.core.groupby.generic.DataFrameGroupBy object at 0x309bc25c0>
- As we've seen already, you should the columns you want to aggregate before using your aggregation method, or you may run into errors!
# This errors, because there are non-numeric columns in penguins
# that it's trying to take the "mean" of, like 'island'.
penguins.groupby('species').mean()
--------------------------------------------------------------------------- TypeError Traceback (most recent call last) File ~/miniforge3/envs/pds/lib/python3.10/site-packages/pandas/core/groupby/groupby.py:1870, in GroupBy._agg_py_fallback(self, how, values, ndim, alt) 1869 try: -> 1870 res_values = self.grouper.agg_series(ser, alt, preserve_dtype=True) 1871 except Exception as err: File ~/miniforge3/envs/pds/lib/python3.10/site-packages/pandas/core/groupby/ops.py:850, in BaseGrouper.agg_series(self, obj, func, preserve_dtype) 848 preserve_dtype = True --> 850 result = self._aggregate_series_pure_python(obj, func) 852 npvalues = lib.maybe_convert_objects(result, try_float=False) File ~/miniforge3/envs/pds/lib/python3.10/site-packages/pandas/core/groupby/ops.py:871, in BaseGrouper._aggregate_series_pure_python(self, obj, func) 870 for i, group in enumerate(splitter): --> 871 res = func(group) 872 res = extract_result(res) File ~/miniforge3/envs/pds/lib/python3.10/site-packages/pandas/core/groupby/groupby.py:2376, in GroupBy.mean.<locals>.<lambda>(x) 2373 else: 2374 result = self._cython_agg_general( 2375 "mean", -> 2376 alt=lambda x: Series(x).mean(numeric_only=numeric_only), 2377 numeric_only=numeric_only, 2378 ) 2379 return result.__finalize__(self.obj, method="groupby") File ~/miniforge3/envs/pds/lib/python3.10/site-packages/pandas/core/series.py:6226, in Series.mean(self, axis, skipna, numeric_only, **kwargs) 6218 @doc(make_doc("mean", ndim=1)) 6219 def mean( 6220 self, (...) 6224 **kwargs, 6225 ): -> 6226 return NDFrame.mean(self, axis, skipna, numeric_only, **kwargs) File ~/miniforge3/envs/pds/lib/python3.10/site-packages/pandas/core/generic.py:11969, in NDFrame.mean(self, axis, skipna, numeric_only, **kwargs) 11962 def mean( 11963 self, 11964 axis: Axis | None = 0, (...) 11967 **kwargs, 11968 ) -> Series | float: > 11969 return self._stat_function( 11970 "mean", nanops.nanmean, axis, skipna, numeric_only, **kwargs 11971 ) File ~/miniforge3/envs/pds/lib/python3.10/site-packages/pandas/core/generic.py:11926, in NDFrame._stat_function(self, name, func, axis, skipna, numeric_only, **kwargs) 11924 validate_bool_kwarg(skipna, "skipna", none_allowed=False) > 11926 return self._reduce( 11927 func, name=name, axis=axis, skipna=skipna, numeric_only=numeric_only 11928 ) File ~/miniforge3/envs/pds/lib/python3.10/site-packages/pandas/core/series.py:6134, in Series._reduce(self, op, name, axis, skipna, numeric_only, filter_type, **kwds) 6130 raise TypeError( 6131 f"Series.{name} does not allow {kwd_name}={numeric_only} " 6132 "with non-numeric dtypes." 6133 ) -> 6134 return op(delegate, skipna=skipna, **kwds) File ~/miniforge3/envs/pds/lib/python3.10/site-packages/pandas/core/nanops.py:147, in bottleneck_switch.__call__.<locals>.f(values, axis, skipna, **kwds) 146 else: --> 147 result = alt(values, axis=axis, skipna=skipna, **kwds) 149 return result File ~/miniforge3/envs/pds/lib/python3.10/site-packages/pandas/core/nanops.py:404, in _datetimelike_compat.<locals>.new_func(values, axis, skipna, mask, **kwargs) 402 mask = isna(values) --> 404 result = func(values, axis=axis, skipna=skipna, mask=mask, **kwargs) 406 if datetimelike: File ~/miniforge3/envs/pds/lib/python3.10/site-packages/pandas/core/nanops.py:720, in nanmean(values, axis, skipna, mask) 719 the_sum = values.sum(axis, dtype=dtype_sum) --> 720 the_sum = _ensure_numeric(the_sum) 722 if axis is not None and getattr(the_sum, "ndim", False): File ~/miniforge3/envs/pds/lib/python3.10/site-packages/pandas/core/nanops.py:1693, in _ensure_numeric(x) 1691 if isinstance(x, str): 1692 # GH#44008, GH#36703 avoid casting e.g. strings to numeric -> 1693 raise TypeError(f"Could not convert string '{x}' to numeric") 1694 try: TypeError: Could not convert string 'TorgersenTorgersenTorgersenTorgersenTorgersenTorgersenTorgersenTorgersenTorgersenTorgersenTorgersenTorgersenTorgersenTorgersenTorgersenBiscoeBiscoeBiscoeBiscoeBiscoeBiscoeBiscoeBiscoeBiscoeBiscoeDreamDreamDreamDreamDreamDreamDreamDreamDreamDreamDreamDreamDreamDreamDreamDreamDreamDreamDreamBiscoeBiscoeBiscoeBiscoeBiscoeBiscoeBiscoeBiscoeBiscoeBiscoeBiscoeBiscoeBiscoeBiscoeBiscoeBiscoeBiscoeBiscoeTorgersenTorgersenTorgersenTorgersenTorgersenTorgersenTorgersenTorgersenTorgersenTorgersenTorgersenTorgersenTorgersenTorgersenTorgersenTorgersenDreamDreamDreamDreamDreamDreamDreamDreamDreamDreamDreamDreamDreamDreamDreamDreamBiscoeBiscoeBiscoeBiscoeBiscoeBiscoeBiscoeBiscoeBiscoeBiscoeBiscoeBiscoeBiscoeBiscoeBiscoeBiscoeTorgersenTorgersenTorgersenTorgersenTorgersenTorgersenTorgersenTorgersenTorgersenTorgersenTorgersenTorgersenTorgersenTorgersenTorgersenTorgersenDreamDreamDreamDreamDreamDreamDreamDreamDreamDreamDreamDreamDreamDreamDreamDreamDreamDreamDreamDream' to numeric The above exception was the direct cause of the following exception: TypeError Traceback (most recent call last) Cell In[72], line 3 1 # This errors, because there are non-numeric columns in penguins 2 # that it's trying to take the "mean" of, like 'island'. ----> 3 penguins.groupby('species').mean() File ~/miniforge3/envs/pds/lib/python3.10/site-packages/pandas/core/groupby/groupby.py:2374, in GroupBy.mean(self, numeric_only, engine, engine_kwargs) 2367 return self._numba_agg_general( 2368 grouped_mean, 2369 executor.float_dtype_mapping, 2370 engine_kwargs, 2371 min_periods=0, 2372 ) 2373 else: -> 2374 result = self._cython_agg_general( 2375 "mean", 2376 alt=lambda x: Series(x).mean(numeric_only=numeric_only), 2377 numeric_only=numeric_only, 2378 ) 2379 return result.__finalize__(self.obj, method="groupby") File ~/miniforge3/envs/pds/lib/python3.10/site-packages/pandas/core/groupby/groupby.py:1925, in GroupBy._cython_agg_general(self, how, alt, numeric_only, min_count, **kwargs) 1922 result = self._agg_py_fallback(how, values, ndim=data.ndim, alt=alt) 1923 return result -> 1925 new_mgr = data.grouped_reduce(array_func) 1926 res = self._wrap_agged_manager(new_mgr) 1927 out = self._wrap_aggregated_output(res) File ~/miniforge3/envs/pds/lib/python3.10/site-packages/pandas/core/internals/managers.py:1428, in BlockManager.grouped_reduce(self, func) 1424 if blk.is_object: 1425 # split on object-dtype blocks bc some columns may raise 1426 # while others do not. 1427 for sb in blk._split(): -> 1428 applied = sb.apply(func) 1429 result_blocks = extend_blocks(applied, result_blocks) 1430 else: File ~/miniforge3/envs/pds/lib/python3.10/site-packages/pandas/core/internals/blocks.py:366, in Block.apply(self, func, **kwargs) 360 @final 361 def apply(self, func, **kwargs) -> list[Block]: 362 """ 363 apply the function to my values; return a block if we are not 364 one 365 """ --> 366 result = func(self.values, **kwargs) 368 result = maybe_coerce_values(result) 369 return self._split_op_result(result) File ~/miniforge3/envs/pds/lib/python3.10/site-packages/pandas/core/groupby/groupby.py:1922, in GroupBy._cython_agg_general.<locals>.array_func(values) 1919 else: 1920 return result -> 1922 result = self._agg_py_fallback(how, values, ndim=data.ndim, alt=alt) 1923 return result File ~/miniforge3/envs/pds/lib/python3.10/site-packages/pandas/core/groupby/groupby.py:1874, in GroupBy._agg_py_fallback(self, how, values, ndim, alt) 1872 msg = f"agg function failed [how->{how},dtype->{ser.dtype}]" 1873 # preserve the kind of exception that raised -> 1874 raise type(err)(msg) from err 1876 if ser.dtype == object: 1877 res_values = res_values.astype(object, copy=False) TypeError: agg function failed [how->mean,dtype->object]
penguins.groupby('species')[['bill_length_mm', 'bill_depth_mm']].mean()
bill_length_mm | bill_depth_mm | |
---|---|---|
species | ||
Adelie | 38.82 | 18.35 |
Chinstrap | 48.83 | 18.42 |
Gentoo | 47.57 | 15.00 |
Aggregation¶
penguins.groupby('species')['bill_length_mm'].mean()
- Once we create a
DataFrameGroupBy
orSeriesGroupBy
object, we need to apply some function to each group, and combine the results.
- The most common operation we apply to each group is an aggregation, but we'll see examples of filtrations and transformations soon
Remember, aggregation is the act of combining many values into a single value.
- To perform an aggregation, use an aggregation method on the
DataFrameGroupBy
orSeriesGroupBy
object, e.g..mean()
,.max()
, or.median()
.
Let's look at some examples.
penguins_small
species | body_mass_g | sex | |
---|---|---|---|
0 | Adelie | 3750.0 | Male |
150 | Chinstrap | 3725.0 | Male |
300 | Gentoo | 4875.0 | Female |
1 | Adelie | 3800.0 | Female |
251 | Gentoo | 4350.0 | Female |
151 | Chinstrap | 3950.0 | Female |
301 | Gentoo | 5550.0 | Male |
penguins_small.groupby('species')['body_mass_g'].mean()
species Adelie 3775.0 Chinstrap 3837.5 Gentoo 4925.0 Name: body_mass_g, dtype: float64
# Note that this worked on the entire DataFrame!
# But, if all we wanted are the sums of `'body_mass_g'
# for each species, this is slower than
# penguins_small.groupby('species')['body_mass_g'].mean().
penguins_small.groupby('species').sum()
body_mass_g | sex | |
---|---|---|
species | ||
Adelie | 7550.0 | MaleFemale |
Chinstrap | 7675.0 | MaleFemale |
Gentoo | 14775.0 | FemaleFemaleMale |
# Often used in conjunction with sort_values.
# Remember this when you work on the activity in a few slides!
penguins_small.groupby('species').last()
body_mass_g | sex | |
---|---|---|
species | ||
Adelie | 3800.0 | Female |
Chinstrap | 3950.0 | Female |
Gentoo | 5550.0 | Male |
penguins_small.groupby('species').max()
body_mass_g | sex | |
---|---|---|
species | ||
Adelie | 3800.0 | Male |
Chinstrap | 3950.0 | Male |
Gentoo | 5550.0 | Male |
Column independence¶
- Within each group, the aggregation method is applied to each column independently.
penguins_small.groupby('species').max()
body_mass_g | sex | |
---|---|---|
species | ||
Adelie | 3800.0 | Male |
Chinstrap | 3950.0 | Male |
Gentoo | 5550.0 | Male |
- The above result is not telling us that there is a
'Male'
'Adelie'
penguin with a'body_mass_g'
of3800.0
!
# This penguin is Female!
penguins_small.loc[(penguins['species'] == 'Adelie') & (penguins['body_mass_g'] == 3800.0)]
species | body_mass_g | sex | |
---|---|---|---|
1 | Adelie | 3800.0 | Female |
Activity
Find the 'species'
, 'island'
, and 'body_mass_g'
of the heaviest 'Male'
and 'Female'
penguins in penguins
(not penguins_small
).
# General idea: Sort the penguibs by mass in decreasing order.
# Then, the first male penguin that appears is the heaviest male penguin,
# and the first female penguin that appears is the heaviest female penguin.
# For each sex, take the first row.
(
penguins
.sort_values('body_mass_g', ascending=False)
.groupby('sex')
.first()
)
species | island | bill_length_mm | bill_depth_mm | flipper_length_mm | body_mass_g | |
---|---|---|---|---|---|---|
sex | ||||||
Female | Gentoo | Biscoe | 45.2 | 14.8 | 212.0 | 5200.0 |
Male | Gentoo | Biscoe | 49.2 | 15.2 | 221.0 | 6300.0 |
Beyond default aggregation methods¶
- There are many built-in aggregation methods.
- What if you want to apply different aggregation methods to different columns?
- What if the aggregation method you want to use doesn't already exist in
pandas
?
The aggregate
method¶
DataFrameGroupBy
andSeriesGroupBy
objects have a generalaggregate
method, which aggregates using one or more operations.
Remember, aggregation is the act of combining many values into a single value.
- There are many ways of using
aggregate
; refer to the documentation for a comprehensive list.
Per the documentation,agg
is an alias foraggregate
.
- Example arguments:
- A single function.
- A list of functions.
- A dictionary mapping column names to functions.
- We've attached a Reference Slide with examples.
- How many penguins are there of each
'species'
, and what is the mean'body_mass_g'
of each'species'
?
(
penguins
.groupby('species')
['body_mass_g']
.aggregate(['count', 'mean'])
)
count | mean | |
---|---|---|
species | ||
Adelie | 146 | 3706.16 |
Chinstrap | 68 | 3733.09 |
Gentoo | 119 | 5092.44 |
- What is the maximum
'bill_length_mm'
of each'species'
, and which'island'
s is each'species'
found on?
(
penguins
.groupby('species')
.agg({'bill_length_mm': 'max', 'island': 'unique'})
)
bill_length_mm | island | |
---|---|---|
species | ||
Adelie | 46.0 | [Torgersen, Biscoe, Dream] |
Chinstrap | 58.0 | [Dream] |
Gentoo | 59.6 | [Biscoe] |
Activity
What is the interquartile range of the 'body_mass_g'
of each 'species'
?
The interquartile range of a distribution is defined as:
$$\text{75th percentile} - \text{25th percentile}$$*Hint*: Use np.percentile
, and pass agg
/aggregate
a custom function.
# Here, the argument to agg is a function,
# which takes in a Series and returns a scalar.
def iqr(s):
return np.percentile(s, 75) - np.percentile(s, 25)
(
penguins
.groupby('species')
['body_mass_g']
.agg(iqr)
)
species Adelie 637.5 Chinstrap 462.5 Gentoo 800.0 Name: body_mass_g, dtype: float64
Question 🤔 (Answer at practicaldsc.org/q)
Remember that you can always ask questions anonymously at the link above!
What questions do you have?
Split-apply-combine, revisited¶
- When we introduced the split-apply-combine pattern, the "apply" step involved aggregation – our final DataFrame had one row for each group.
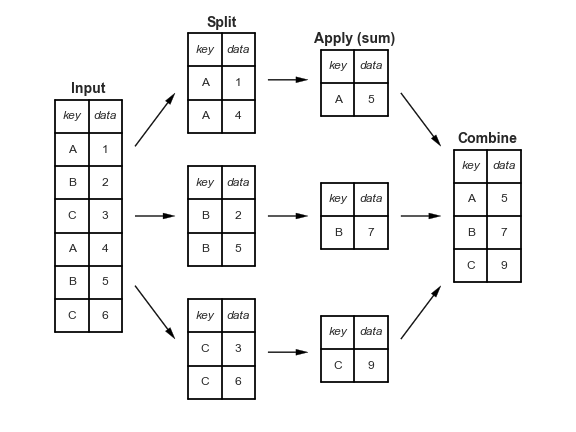
- Instead of aggregating during the apply step, we could instead perform a filtration, in which we keep only the groups that satisfy some condition.
- Or a transformation, in which we perform operations to every value within each group.
Grouping, then filtering¶
- To keep only the groups that satisfy a particular condition, use the
filter
method on aDataFrameGroupBy
/SeriesGroupBy
object.
Thefilter
method takes in a function, which itself takes in a DataFrame/Series and return a single Boolean. The result is a new DataFrame/Series with only the groups for which the filter function returnedTrue
.
- For example, suppose we want only the
'species'
whose average'bill_length_mm'
is above 39.
(
penguins
.groupby('species')
.filter(lambda df: df['bill_length_mm'].mean() > 39)
)
species | island | bill_length_mm | bill_depth_mm | flipper_length_mm | body_mass_g | sex | |
---|---|---|---|---|---|---|---|
146 | Chinstrap | Dream | 46.5 | 17.9 | 192.0 | 3500.0 | Female |
147 | Chinstrap | Dream | 50.0 | 19.5 | 196.0 | 3900.0 | Male |
148 | Chinstrap | Dream | 51.3 | 19.2 | 193.0 | 3650.0 | Male |
... | ... | ... | ... | ... | ... | ... | ... |
330 | Gentoo | Biscoe | 50.4 | 15.7 | 222.0 | 5750.0 | Male |
331 | Gentoo | Biscoe | 45.2 | 14.8 | 212.0 | 5200.0 | Female |
332 | Gentoo | Biscoe | 49.9 | 16.1 | 213.0 | 5400.0 | Male |
187 rows × 7 columns
- No more
'Adelie'
s!
Activity
Create a new DataFrame with only the rows in penguins
for popular 'species'
– that is, 'species'
with at least 100 penguins.
(
penguins
.groupby('species')
.filter(lambda df: df.shape[0] >= 100)
)
species | island | bill_length_mm | bill_depth_mm | flipper_length_mm | body_mass_g | sex | |
---|---|---|---|---|---|---|---|
0 | Adelie | Torgersen | 39.1 | 18.7 | 181.0 | 3750.0 | Male |
1 | Adelie | Torgersen | 39.5 | 17.4 | 186.0 | 3800.0 | Female |
2 | Adelie | Torgersen | 40.3 | 18.0 | 195.0 | 3250.0 | Female |
... | ... | ... | ... | ... | ... | ... | ... |
330 | Gentoo | Biscoe | 50.4 | 15.7 | 222.0 | 5750.0 | Male |
331 | Gentoo | Biscoe | 45.2 | 14.8 | 212.0 | 5200.0 | Female |
332 | Gentoo | Biscoe | 49.9 | 16.1 | 213.0 | 5400.0 | Male |
265 rows × 7 columns
# Note that to just find the 'species' with at least 100 penguins,
# we didn't need to group:
penguins['species'].value_counts()
species Adelie 146 Gentoo 119 Chinstrap 68 Name: count, dtype: int64
- Suppose we want to convert the
'body_mass_g'
column to to z-scores (i.e. standard units):
def z_score(x):
return (x - x.mean()) / x.std(ddof=0)
z_score(penguins['body_mass_g'])
0 -0.57 1 -0.51 2 -1.19 ... 330 1.92 331 1.23 332 1.48 Name: body_mass_g, Length: 333, dtype: float64
- Now, what if we wanted the z-score within each group?
- To do so, we can use the
transform
method on aDataFrameGroupBy
object. Thetransform
method takes in a function, which itself takes in a Series and returns a new Series.
- A transformation produces a DataFrame or Series of the same size – it is not an aggregation!
z_mass = (penguins
.groupby('species')
['body_mass_g']
.transform(z_score))
z_mass
0 0.10 1 0.21 2 -1.00 ... 330 1.32 331 0.22 332 0.62 Name: body_mass_g, Length: 333, dtype: float64
penguins.assign(z_mass=z_mass)
species | island | bill_length_mm | bill_depth_mm | flipper_length_mm | body_mass_g | sex | z_mass | |
---|---|---|---|---|---|---|---|---|
0 | Adelie | Torgersen | 39.1 | 18.7 | 181.0 | 3750.0 | Male | 0.10 |
1 | Adelie | Torgersen | 39.5 | 17.4 | 186.0 | 3800.0 | Female | 0.21 |
2 | Adelie | Torgersen | 40.3 | 18.0 | 195.0 | 3250.0 | Female | -1.00 |
... | ... | ... | ... | ... | ... | ... | ... | ... |
330 | Gentoo | Biscoe | 50.4 | 15.7 | 222.0 | 5750.0 | Male | 1.32 |
331 | Gentoo | Biscoe | 45.2 | 14.8 | 212.0 | 5200.0 | Female | 0.22 |
332 | Gentoo | Biscoe | 49.9 | 16.1 | 213.0 | 5400.0 | Male | 0.62 |
333 rows × 8 columns
display_df(penguins.assign(z_mass=z_mass), rows=8)
species | island | bill_length_mm | bill_depth_mm | flipper_length_mm | body_mass_g | sex | z_mass | |
---|---|---|---|---|---|---|---|---|
0 | Adelie | Torgersen | 39.1 | 18.7 | 181.0 | 3750.0 | Male | 0.10 |
1 | Adelie | Torgersen | 39.5 | 17.4 | 186.0 | 3800.0 | Female | 0.21 |
2 | Adelie | Torgersen | 40.3 | 18.0 | 195.0 | 3250.0 | Female | -1.00 |
3 | Adelie | Torgersen | 36.7 | 19.3 | 193.0 | 3450.0 | Female | -0.56 |
... | ... | ... | ... | ... | ... | ... | ... | ... |
329 | Gentoo | Biscoe | 46.8 | 14.3 | 215.0 | 4850.0 | Female | -0.49 |
330 | Gentoo | Biscoe | 50.4 | 15.7 | 222.0 | 5750.0 | Male | 1.32 |
331 | Gentoo | Biscoe | 45.2 | 14.8 | 212.0 | 5200.0 | Female | 0.22 |
332 | Gentoo | Biscoe | 49.9 | 16.1 | 213.0 | 5400.0 | Male | 0.62 |
333 rows × 8 columns
- Note that above, penguin 340 has a larger
'body_mass_g'
than penguin 0, but a lower'z_mass'
.- Penguin 0 has an above average
'body_mass_g'
among'Adelie'
penguins. - Penguin 340 has a below average
'body_mass_g'
among'Gentoo'
penguins. Remember from earlier that the average'body_mass_g'
of'Gentoo'
penguins is much higher than for other species.
- Penguin 0 has an above average
What's next?¶
- Can we group on multiple columns at once?
- What does
pivot_table
do?
- How do we combine two DataFrames with information about similar individuals?
- How do we deal with missing values?
- How do we decide which type of visualization to create?